Digital Transformation (DX), already a trend in higher education, became a sector-wide imperative during the onset of the pandemic when almost all functions (instruction and support) either went remote or went away. The acceleration of DX was a temporary solution for a temporary problem, and it might now be expected to slow in the post-pandemic era, but the likelihood of an actual reversal (back to analog) is near zero given the innumerable advantages of ed tech. So now, nearly all institutions are using ed tech, and almost all ed tech uses AI.
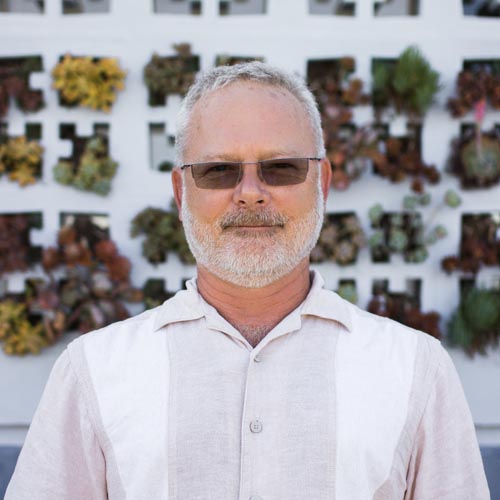
DX is a heavy lift for any institution, but most are also struggling with other interconnected issues, most prominently centered on Diversity-Equity-Inclusion (DEI). For example, widespread student disengagement is strongest among under-served populations. That further worsens the long-term enrollment decline, which was already headed toward a metaphorical ‘demographic cliff’ even before the pandemic stop-outs and recruit-hungry job market. That decline has been steepest for community colleges, which enroll disproportionately more minorities than other market segments. Additionally, they, with the other perennially underfunded Minority Serving Institutions (MSIs), are now facing a pandemic-driven recession alongside rising inflation. All of this contributes to the stagnation of transfer outcomes, making it harder for college students to earn bachelor’s degrees at universities, further widening the equity gap.
Given the ubiquity of AI, and the strong correlations between the DEI problems and all the rest, the Law of the Instrument seemingly makes the next step along the path quite clear: We could implement ed tech with more AI – virtual learning environments, chatbots, predictive analytics, machine learning, etc. to compensate for staffing shortages, re-engage disengaged students and analyze student data to optimize enrollment/retention/completion/transfer outcomes. But keep in mind this is the same AI that has repeatedly shown itself to perpetuate bias by consistently steering minorities and nontraditional students away from STEM majors and disqualifying candidates from tech jobs due to their past education and socioeconomic status. In addition, it has inadvertently incentivized direct abuse by its end-users (for instance, the ‘Caucasian-Only’ setting for an AI-powered recruitment tool, or the ‘Risk’ predictors that steer Black students away from math/science programs in AI-powered advising software).
AI-powered ed tech needs more scrutiny but advocating for more direct human oversight on algorithmic decision-making would add further cost to something presumably adopted as a ‘cost saver’ and it runs counter to the notion of AI being ‘automated and autonomous’. Also, because this is ‘year 0’ of the ‘new normal’ of the post-pandemic era, an argument can be made that no reliable historical data exists for training the predictive-analytics algorithms themselves, and research shows that human oversight of the outputs of these algorithms is generally ineffective, further compounding the risks and expenditures.
More work is needed on how AI-powered ed tech is built, but it is also critical to focus on what the actual DEI problem is and what is required to solve it. AI itself can’t be biased, and AI certainly isn’t objectively recognizing some real-world demographic stratification of abilities. But AI does orient itself from default norms that can themselves be biased, and human software builders introduce unrecognized biases of their own, so there is seemingly no end of ‘tweaking’ needed to obtain desired outcomes from AI.
Diversity matters: Why change is vital in university business leadership
The solution? Tackle the problem at its source. Endlessly refining the technology should not be prioritized over addressing the DEI problem that the technology was ostensibly introduced to address. Under-resourced institutions might better serve the under-served by channeling those limited resources into building DEI cultures among the people who make, and the people who make use of, those tools.
AI can be built to address DEI (for example, a recent grant was awarded at UNR to build a gaming tool to coach faculty on appropriate responses to DEI issues on campus). But it is also important to recognize that the more we focus on improving AI, the more we drain resources that could go toward more traditional and direct solutions for inequity in transfer and completion outcomes. This includes increasing the advisor-to-student ratio, creating and storing course-to-course equivalencies or conducting diversity training. Of course, the stock response is that such ‘legacy’ solutions no longer work, but are we certain that it isn’t just a self-fulfilling prophecy if those solutions are deprioritized and defunded in favor of endlessly tinkering with AI?
Why are we introducing AI to replace existing solutions, especially if the AI tends to perpetuate the very norms that so many are trying to escape? And if the broader culture cannot agree on the extent to which inequity is a ‘systemic’ problem, then won’t AI continue to reflect a patchwork of existing localized biases, even after the tinkering is complete? For example, suppose we automate credit evaluation via an automated course recommendation system plus a chatbot: Course-to-course equivalency decisions will still be made by humans and those will still require human quality control and periodic review to ensure consistency and fairness. But transfer outcomes will not change unless there are changes to the standards and norms upon which those equivalency decisions are based. Meanwhile, human transcript evaluators and advisors will continue to be available, but now via third-party consultancies and only for those who can pay, thus exacerbating stratification.
AI algorithms have not been shown to make predictions that are vastly better than humans; they just make those decisions much more rapidly and efficiently. Because humans are incapable of performing effective oversight for the output of those algorithms, even a ‘transparent’ AI solution is de facto a ‘black box’ in terms of steering outcomes toward policy goals like DEI. Nevertheless, AI technology is here to stay, and so it must be updated to fit today’s society and ensure that DEI efforts are prioritized to give all students, especially historically under-served students, the same opportunities and resources needed to succeed. But if we continue to overcommit to AI, it may be time to recognize that we may be shepherding scarce resources toward simply maintaining the status quo – just with shinier tools.
As Senior Market Analyst for CollegeSource, Stan Novak researches and identifies postsecondary education landscape trends and relationships among institutions and students to help guide the future direction of product innovation and market strategies for the company. Stan has worked with CollegeSource since 2003.
More from UB