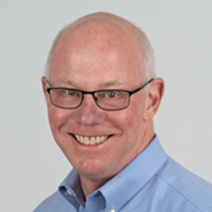
The COVID-19 pandemic dramatically accelerated higher education’s move away from including standardized test scores in the admissions processes. In 2019, about 1,070 institutions were “test-optional,” but by this fall the number swelled to more than 1,800. This past year, 89 percent of Common App members did not require test scores, compared to just one third the previous year.
Even before pandemic-fueled test cancellations and postponements necessitated the change, institutions were exploring the potential of test-optional policies as a way of increasing access, particularly for students from underrepresented and underserved populations.
For decades, research has indicated standardized tests were biased against students from certain populations. Critics have pointed out that students from higher income families benefit from better schools, access to test preparation services, and the ability to take the test many times—helping to guarantee higher scores that attract the attention of selective institutions.
But early research shows going test-optional may not be the boon for diversity and equity some had hoped. One study of 100 private colleges and universities found that test-optional admissions increased the share of Black, Latino, and Native American students by just one percentage point. The share of Pell eligible students from low-income backgrounds increased by only 1 percentage point.
Test scores have been a foundational component of the admission process for the vast majority of institutions for decades. Test-optional has now hit the mainstream—so now what? If that data source is no longer available, how will institutions know which admitted students are good fits and present the academic potential to persist and graduate?
Removing a perceived barrier to college access for students is a step in the right direction, to be sure. But the jury will be out until we can research the impact of test-optional policies among public institutions, which enroll the bulk of the students in the United States.
To make a true transformational move away from test scores, that is also sustainable, institutions must improve the ways in which they leverage the extensive data they obtain about a student during the admissions process.
Test-optional policies should be paired with advanced analytics to capture a prospective student’s life experiences more fully, how the student will likely fit within an institution’s culture, and their likelihood to persist and graduate. The reality is that admissions and enrollment management professionals already can, and do, use a range of data inputs and models to build their classes—often in highly-sophisticated ways.
As higher education shifts away from standardized college entrance exams as a heavily-weighted proxy for college readiness, the urgency of finding new ways to identify and evaluate prospective students will only continue to increase. Emerging technologies now allow—with a greater degree of precision—institutions to shape a class that includes students from a diverse set of backgrounds but that is still consistent with their missions, visions, and values.
There are many variables and criteria besides testing on which institutions can rely, including grade point average, class rank, extracurricular activities, and honor courses. When combined with other data points such as socioeconomic status, projected major, and high school of origin, a more granular view of the individual student—and cohorts of prospective students—emerges.
Institutions that go test-optional should revise their admissions guidelines based on these metrics. Using historical data, and excluding test scores, institutions can create a model to determine which students in the past would still have been admitted using this new rubric. This provides admissions teams with a better-informed starting point for their models moving forward—one that allows them to take into account a wide variety of factors beyond test scores.
When paired with a stronger focus on data and predictive modeling, the revised guidelines will help institutions determine which students might be a good fit and also provide predictions of student success. Institutions can, for example, mine admitted student data to understand which interventions a student may need to succeed and apply those interventions before or soon after a student starts their first semester.
Moving to test-optional admissions creates additional complexity in developing a financial aid leveraging model. For example, many institutions have a defined matrix in place for administering financial aid, particularly merit-based aid, typically relying on factors such as test scores, class rank, GPA, and other academic indicators. The absence of test scores adds a new level of uncertainty in developing the financial aid matrix and determining the amount of aid students who apply test-optional should receive if admitted.
Developing a matrix is complicated by an institution’s varied enrollment goal, such as maximizing net tuition revenue, academic profile, diversity, and residency—goals that often conflict with one another. A robust, data-informed approach to test-optional admissions can give institutions a better understanding of financial aid sensitivity, from a macro-level view of the entire student population to the specific needs of individual students.
The application of analytical models can provide incomparable insights to the enrollment management leader and institution—highlighting the limitations and tradeoffs associated with achieving multiple enrollment goals. This process allows the institution to optimize financial aid resources in achieving their enrollment goals.
This is not to say institutions should only admit those students the model predicts will be successful. Nor is it to say that data and predictive modeling should be viewed as an infallible crystal ball determining students’ futures. Analytic models inform admissions decision-making, but the human element is still critical to ensure the institution admits students consistent with its values.
A recent Brookings Institution report cautioned—with good reason—about the risks associated with using predictive modeling as a blunt instrument. New data models can never replace the human insight; care and judgment required to understand the unique merits and potential of each student, and consider those against the institution’s values, mission, and vision.
What advanced analytics can provide is a more nuanced understanding of the student and their ability to be successful at an institution, the support that they may need—helping the enrollment leader to manage financial aid investments more effectively. For instance, predictive modeling provides institutions with insights into which students may need additional support, allowing campuses to diversify their incoming classes while providing all students with the support needed to succeed starting as early as orientation.
Going test-optional is only the beginning for institutions looking to create a more equitable system of college admissions. With the hindsight of recent experience, the shift to test-optional in and of itself was accomplished with speed and decisiveness that very few would have predicted.
The more important—and vexing—task for colleges and universities now is to build a system that can break down barriers to college access for historically underrepresented students—while ensuring that students are set up for success before and after they arrive on campus.
Chris Lucier is the Director of Partner Relationships at Othot, leveraging over 20 years of experience in admissions and strategic enrollment management to help colleges and universities adopt data-driven decision making in addressing enrollment and students success challenges.
More from UB